AI agents significantly improve task automation and decision-making by functioning autonomously across various complexities, from reactive to multi-agent systems. The concepts of Agentic Workflow and Agentic AI emphasize their capacity for independent task management, seamless integration with human processes, and scalable efficiency, contributing to enhanced operational productivity and innovation in business environments.
The advent of artificial intelligence (AI) has revolutionized how we approach problem-solving and task execution across various sectors. Among the most impactful innovations are AI agents, intelligent software systems designed to operate autonomously or collaboratively, significantly enhancing productivity and decision-making. This blog explores the various types of AI agents, their mechanisms of operation, and a comparative analysis of co-pilots, AI agents, and AI teammates.
Furthermore, it delves into the concepts of agentic workflow and agentic AI, emphasizing how these technologies can be integrated into organizational processes to optimize efficiency and effectiveness. As AI continues to evolve, understanding its fundamental components and functions is crucial for leveraging its potential in modern business landscapes.
What is AI Agent?
AI Agents are software programs that use artificial intelligence to perform tasks independently, without human intervention. They are designed to understand and process data, make decisions, and execute actions based on predefined rules or learned behaviors. These agents can function in various environments, from simple applications to complex systems, providing solutions for routine task automation and advanced problem-solving.
AI Agents gather information through sensors or data inputs, analyze and interpret this data using reasoning algorithms, and then make decisions on actions to take. This process involves understanding context, identifying patterns, and determining the best course of action. Finally, they execute these actions, whether it’s responding to a user query, making recommendations, or performing physical tasks.
Example: AI agents include chatbots and virtual assistants like Siri and Alexa, which help users perform tasks and answer questions. In finance, AI agents analyze market trends and execute trades. In healthcare, they assist in diagnosing diseases and suggesting treatments. In customer service, AI agents handle inquiries and provide support, allowing human agents to focus on more complex issues.
Types of AI agents
1. Reactive Agents: Reactive agents operate based on predefined rules and do not have the ability to learn or adapt from past experiences. They react to specific stimuli in their environment and perform actions accordingly. These agents are suitable for tasks that require a straightforward response to inputs. For example, a thermostat adjusting the temperature based on predefined settings is a reactive agent.
2. Deliberative Agents: Deliberative agents possess the ability to reason and plan their actions based on an internal model of the world. They analyze various possibilities and choose the most appropriate course of action. These agents are used in applications requiring more complex decision-making, such as autonomous vehicles and robotic systems.
3. Learning Agents: Learning agents can adapt and improve their performance over time by learning from past experiences. They use machine learning techniques to analyze data, identify patterns, and refine their actions. These agents are valuable in dynamic environments where conditions change frequently. Examples include recommendation systems and predictive analytics tools.
4. Collaborative Agents: Collaborative agents work alongside human users or other AI agents to achieve common goals. They can communicate, share information, and coordinate actions to enhance overall performance. In customer service, collaborative agents assist human agents by providing relevant information and automating routine tasks.
5. Multi-Agent Systems: Multi-agent systems consist of multiple AI agents that interact and collaborate to solve complex problems. Each agent has a specific role and can communicate with others to share knowledge and coordinate actions. These systems are used in areas like supply chain management and distributed computing.
Multi-Agent in Action
The Multi-Agent Architecture coordinates specialized agents to execute tasks like data retrieval, content generation, and visual analysis, managed by an orchestrator. This system efficiently processes user inputs and delivers results through a unified interface.
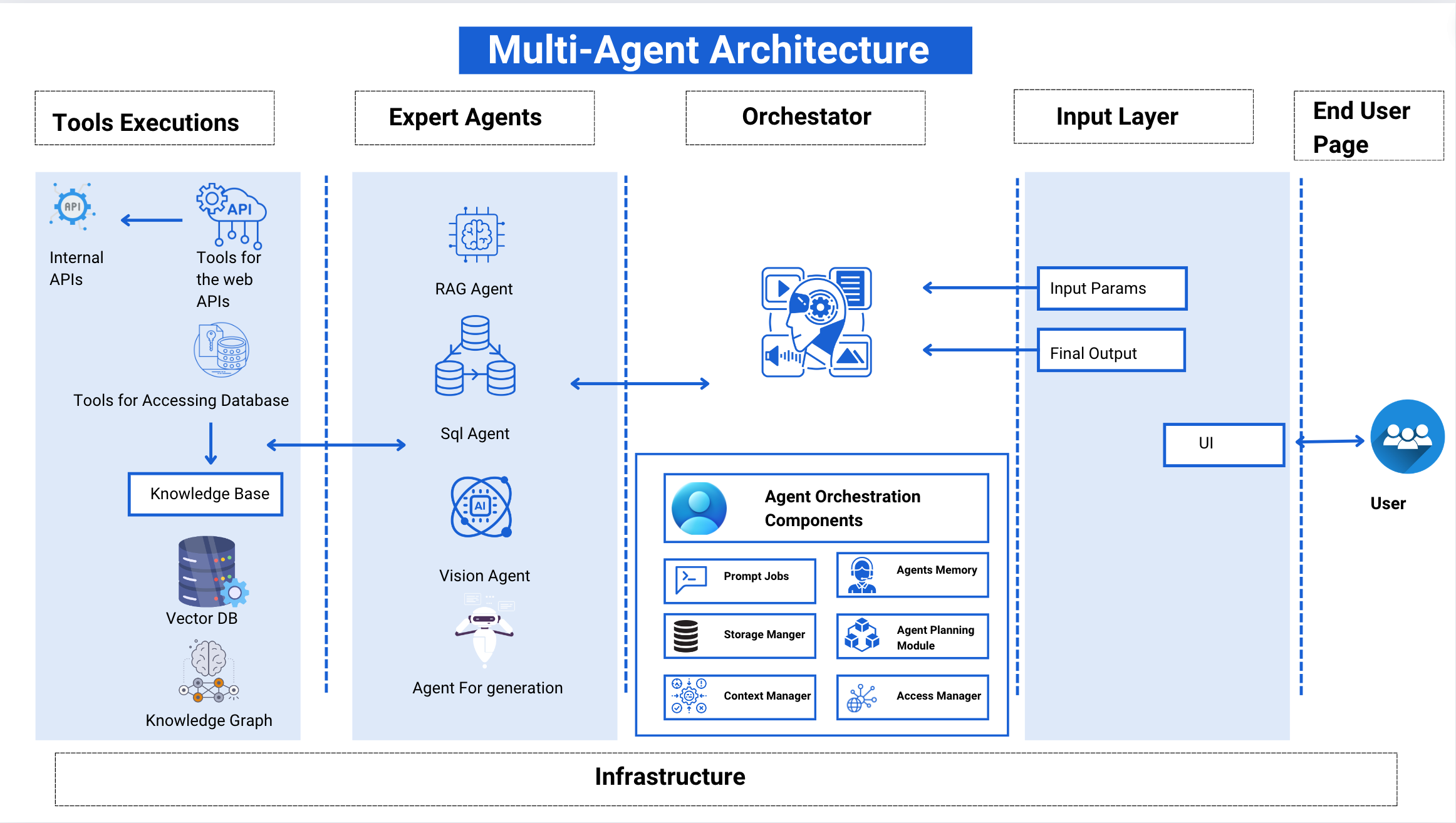
Step 1: Tools Executions
The Tools Executions phase involves the various resources and methods the system uses to gather and process information. This includes internal APIs that help different software components within the system communicate, and tools for accessing web APIs to fetch data from the internet. Additionally, tools are employed to access and retrieve information from databases. The system also utilizes a Knowledge Base to store and retrieve important information, a Vector DB to handle data stored in numerical vectors, and a Knowledge Graph to represent and connect data points and their relationships.
Step 2: Expert Agents
In the Expert Agents phase, specialized agents perform specific tasks to process and analyze data. The RAG Agent retrieves relevant information from the knowledge base or the web and generates responses based on that information. The SQL Agent manages database queries to extract data using SQL. The Vision Agent processes visual inputs like images or videos to extract useful information. Lastly, the Agent for Generation creates content or responses based on the processed data.
Step 3: Orchestrator
The Orchestrator manages and coordinates the activities of different agents. It involves several components: Prompt Jobs to outline tasks for the agents, Agents Memory to store information for future reference, Storage Manager to handle data storage, Agent Planning Module to plan and direct the agents’ activities, Context Manager to track the context of operations, and Access Manager to control access to various resources.
Step 4: Input Layer
The Input Layer handles the data provided by the user. It includes Input Params, which are the parameters or data entered by the user, and the Final Output, which is the information or result the system provides back to the user after processing.
Step 5: End User Page
The End User Page is where the user interacts with the system. It features the User Interface (UI), through which users input their queries or data and receive the system’s responses. The user engages with the UI to facilitate interaction with the system and obtain the final output.
Comparison between CO-Pilots, AI Agents and AI teammates
Feature | Co-Pilots (Assisted Intelligence) | AI Agents (Autonomous Intelligence) | AI Teammates (Collaborative Intelligence) |
Primary Function | Provides support and recommendations to enhance task execution. | Executes specific tasks independently of human input. | Collaborates with humans to achieve mutual objectives and enhance overall performance. |
Interaction with Humans | Offers contextual assistance and adapts based on user input. | Operates autonomously with minimal or no human interaction. | Engages dynamically with humans, adjusting to feedback and contributing to team efforts. |
Task Execution | Enhances human performance by supporting and streamlining task execution. | Handles specific tasks autonomously, focusing on efficiency and consistency. | Partners with humans to tackle complex tasks and projects, facilitating higher-level collaboration. |
Scope of Tasks | Primarily assists with supportive and decision-making tasks. | Focuses on executing well-defined, routine tasks. | Manages a broad range of dynamic and complex tasks, contributing significantly to project outcomes. |
Collaboration | Supports human efforts without being deeply integrated into team workflows. | Operates independently with limited collaborative functions. | Integral to team processes, actively contributing to and enhancing collaborative efforts. |
Human Enhancement | Augments human capabilities through direct support and real-time assistance. | Frees human resources by autonomously handling specific tasks. | Elevates human performance by working in tandem with team members to achieve superior results. |
Agentic Workflow and Agentic AI
Agentic Workflow and Agentic AI are concepts related to the integration and functioning of artificial intelligence systems in complex business processes and decision-making. Here’s an explanation of these concepts:
Agentic Workflow
Agentic Workflow refers to the structured sequence of tasks and decision-making processes managed by AI agents within an organization. This workflow is designed to optimize how AI agents interact with each other and with human operators to accomplish specific business objectives. Key elements of an agentic workflow include:
- 1.Autonomy and Coordination: AI agents operate with a degree of autonomy to perform tasks, but they are also coordinated to ensure that their actions align with organizational goals. This involves setting up systems where agents can work independently while integrating their outputs effectively with other workflows and human inputs.
- 2.Task Management: The workflow defines how tasks are assigned to different AI agents based on their capabilities and roles. This might involve routing tasks to the most suitable agent, monitoring their progress, and adjusting as necessary.
- 3.Decision-Making: Agents make decisions based on predefined rules, machine learning models, or real-time data inputs. The workflow ensures that decision-making processes are streamlined and that agents can act on data efficiently.
- 4.Integration: The workflow integrates AI agents with existing systems and human processes. This ensures that the outputs of AI agents are usable and actionable within the broader context of business operations.
- 5.Efficiency and Optimization: The agentic workflow aims to maximize efficiency by automating repetitive tasks, improving accuracy, and reducing the time required to complete complex processes.
Agentic AI
Agentic AI refers to artificial intelligence systems designed with the capability to operate autonomously and make decisions in a manner similar to humans. The term “agentic” emphasizes the ability of AI to act with agency — meaning it can make decisions, take actions, and influence outcomes independently. Key features of agentic AI include:
- 1.Autonomy: Agentic AI operates independently of human intervention for specific tasks. It can execute actions, make decisions, and adjust its behavior based on real-time data and predefined rules or algorithms.
- 2.Decision-Making: These AI systems are capable of complex decision-making, using data-driven insights and machine learning models. They can evaluate options, predict outcomes, and choose actions that align with their objectives.
- 3.Self-Management: Agentic AI can manage its own processes and adapt to changing conditions without constant human oversight. This includes learning from interactions and optimizing its performance over time.
- 4.Integration: While autonomous, agentic AI systems are designed to integrate seamlessly with existing business processes and technology stacks. They work in conjunction with other systems and human operators to achieve broader organizational goals.
- 5.Scalability: The autonomous nature of agentic AI allows for scalability in handling large volumes of data and tasks. This is particularly useful in environments requiring real-time processing and decision-making across extensive operations.
Conclusion
AI agents are transforming the way tasks are executed in various industries by operating autonomously, collaborating with humans, and improving efficiency across workflows. From reactive agents that follow predefined rules to multi-agent systems solving complex problems, each type of AI agent plays a crucial role in automating processes and enhancing decision-making. The comparison highlights the assistance AI offers — from supporting human activities to acting as full-fledged team members. As AI continues to evolve, its ability to operate independently, adapt to changing conditions, and scale efficiently will significantly influence the future of work and innovation.