Transaction reconciliation streamlines the comparison of financial records, ensuring consistency between internal and external data sources. This process identifies and resolves discrepancies to maintain accuracy, accountability, and compliance within financial operations. By enhancing speed and reducing errors, reconciliation allows finance teams to efficiently manage growing transaction volumes and focus on more strategic activities.
Transaction reconciliation is a cornerstone of financial management, ensuring accuracy across accounts and identifying discrepancies. Traditionally, this process has been time-consuming, error-prone, and reliant on manual interventions. However, the advent of AI-powered agents has redefined how reconciliation tasks are approached in finance. These agents automate complex workflows, analyze large datasets in real-time, and streamline the resolution of mismatched records.
By integrating Agentic AI, businesses can achieve faster, more precise reconciliations, freeing up teams to focus on strategic decision-making. From minimizing errors to enhancing compliance, AI agents are revolutionizing financial processes to meet the demands of a rapidly evolving industry.
What is the Transaction Reconciliation Process?
Transaction reconciliation is the process of comparing and matching internal financial records, such as accounting books and ledgers, with external documents like bank statements or vendor invoices. The primary goal is to identify discrepancies between the two sources, ensuring that all financial transactions are accurately recorded. This process is essential for maintaining the accuracy and integrity of an organization’s financial statements, preventing fraud, and ensuring compliance with regulations.
However, with increasing transaction volumes, businesses are adopting more efficient systems to streamline the process. Regular reconciliation also prepares organizations for audits and mitigates the risks associated with financial inaccuracies, making it a crucial practice for any business operation.
A Brief Overview of Transaction Reconciliation Process in Finance with AI Agents
The transaction reconciliation process in finance involves verifying that financial records match between internal and external sources. Traditionally, this process is time-consuming and prone to human error, often requiring extensive manual intervention. However, the integration of AI agents has significantly transformed this landscape.
AI agents automate many aspects of the reconciliation process by analyzing large volumes of data quickly and accurately. These intelligent systems can detect discrepancies between internal and external records in real-time, flagging any mismatches for review. AI agents also offer enhanced capabilities in matching transactions, identifying patterns, and predicting potential issues before they arise. By automating these tasks, AI Teammates not only reduce human errors but also speed up the reconciliation process, allowing businesses to focus on more strategic activities. This level of automation improves operational efficiency, ensures accuracy, and supports compliance with financial regulations, ultimately leading to better financial decision-making.
Traditional vs. Agentic AI Transaction Reconciliation Process
Aspect | Traditional Transaction Reconciliation | Transaction Reconciliation with AI Agents |
Error Rate | Higher potential for human error | Significantly reduced error rate |
Scalability | Limited scalability | Highly scalable across vast datasets |
Human Involvement | High level of manual intervention | Minimal manual intervention |
Data Processing Capacity | Limited | Processes large volumes of data efficiently |
Anomaly Detection | Reactive and manual | Proactive, automated anomaly detection |
Cost Efficiency | High operational costs | Reduced costs due to automation |
Insight and Analytics | Limited analytics capability | Provides real-time insights and detailed analytics |
Adaptability | Rigid, dependent on predefined rules | Adaptive, learns, and improves over time |
Agentic AI Multi-Agent in Action
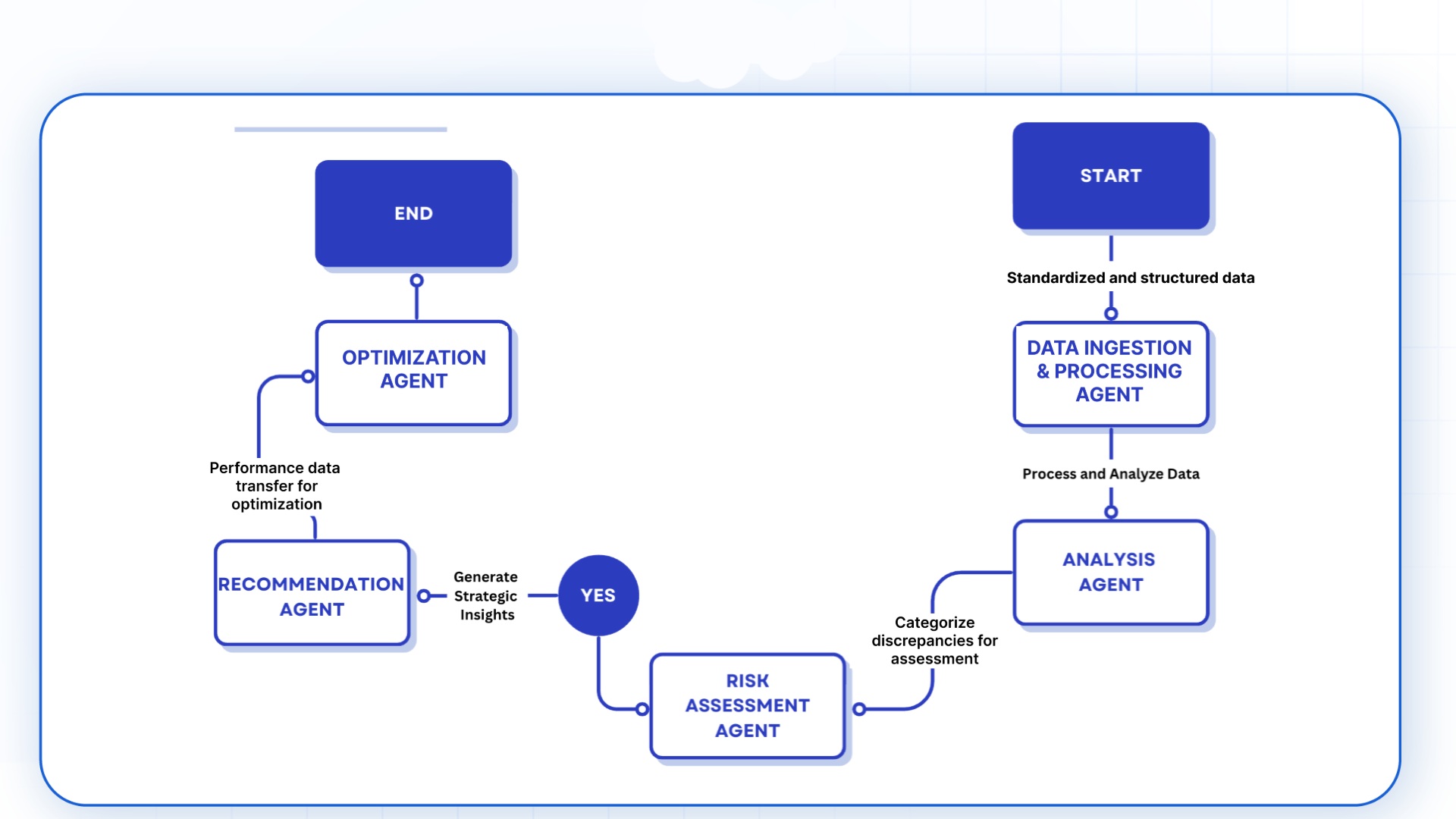
Agentic AI’s multiagent architecture is designed to streamline and automate the complex transaction reconciliation process in finance. By deploying specialized agents, this system can handle high volumes of transactions, detect discrepancies, and resolve issues more efficiently than traditional methods. Below, we explore the agents involved in the process, their roles, and how they work together to achieve seamless reconciliation.
- Data Ingestion and Standardization: The Ingestion Agent automatically gathers transaction data from multiple sources, such as bank statements and invoices, and standardizes the information to ensure consistency, eliminating manual data handling.
- Data Preprocessing and Validation: The Preprocessing Agent ensures that only accurate, high-quality data enters the system by checking for missing values, correcting formats, and applying transformation rules, minimizing errors in subsequent steps.
- Anomaly Detection and Analysis: The Analytics Agent uses advanced algorithms to detect discrepancies, such as duplicate transactions or suspicious activities, in real-time. This proactive detection streamlines the reconciliation process and reduces errors.
- Routing and Case Management: The Routing Agent directs flagged discrepancies to the appropriate team or agent based on the severity of the issue, ensuring that minor problems are resolved quickly, while complex issues are escalated.
- Continuous Monitoring and Optimization: The Monitoring Agent tracks performance across all agents, identifying potential inefficiencies or bottlenecks. The Optimization Agent then adjusts resources and workflows, ensuring the reconciliation process remains scalable and efficient.
Use Cases of Transaction Reconciliation Process in Finance
- Bank Reconciliation: AI agents streamline bank reconciliation by matching bank statements with internal records in real-time, identifying discrepancies instantly, and notifying finance teams. This reduces manual effort and ensures accuracy.
- Credit Card Transaction Reconciliation: For organizations managing multiple credit card transactions, AI agents reconcile transaction data from credit card statements with internal records, detecting any anomalies or unauthorized spending.
- Intercompany Reconciliation: Large organizations with multiple subsidiaries often struggle with intercompany reconciliations. AI agents automate the matching of intercompany transactions, ensuring consistency across financial records.
- Fraud Detection and Anomaly Monitoring: AI agents continuously monitor transaction patterns and flag suspicious activities in real-time, helping to identify potential fraud early and protect the organization from financial loss.
- Regulatory Compliance and Audit Support: By maintaining a clear record of reconciliations and automatically generating audit trails, AI agents support regulatory compliance and simplify audit processes.
The Operational Benefits of the Transaction Reconciliation Process
Implementing AI agents in the transaction reconciliation process yields a range of operational benefits, including:
- Increased Speed and Efficiency: By automating repetitive tasks, reconciliation processes that once took days are now completed in minutes. This allows finance teams to keep pace with real-time transactions and make more timely decisions.
- Error Reduction: Automation helps reduce human errors in data matching, anomaly detection, and report generation. This leads to more accurate financial records, which are crucial for maintaining regulatory compliance and trust.
- Scalability and Flexibility: Automated systems are capable of handling large volumes of transactions across multiple accounts and systems, making them highly scalable and adaptable to growing businesses without requiring additional manual resources.
- Cost Savings: Automating manual processes reduces the need for extensive human resources, resulting in significant savings. This allows companies to reallocate resources to more strategic tasks, further boosting operational efficiency.
- Real-Time Insights and Analytics: Automated systems provide real-time insights into transaction flows, enabling finance teams to promptly identify patterns, trends, and potential risks. This helps in making proactive decisions to mitigate issues before they escalate.
Technologies Transforming Transaction Reconciliation Process
- Natural Language Processing (NLP): NLP helps AI agents understand and process textual information from invoices, bank statements, and other financial documents, making it easier to match records across diverse formats.
- Anomaly Detection Algorithms: Algorithms designed to detect outliers and unusual patterns in transactions help identify discrepancies and potential fraud quickly.
- Predictive Analytics: Predictive analytics enables AI agents to identify patterns in transaction data, anticipate potential issues, and provide insights for more informed decision-making.
- Blockchain for Audit Trails: Blockchain technology ensures a secure, immutable audit trail for reconciliation records, enhancing transparency and accountability.
- Cloud Computing and Data Integration: Cloud-based solutions enable seamless data integration from various sources, making it easier to centralize and reconcile transactions in real time.
The Future Trends of AI Agents for the Transaction Reconciliation Process
- Full Automation of Reconciliation Processes: In the future, advanced automation will handle the entire reconciliation process independently, reducing the need for human intervention and increasing overall accuracy and efficiency.
- Integration with Advanced Analytics: Automation will become increasingly integrated with advanced analytics platforms, offering real-time, data-driven insights into transaction flows and reconciliation processes, which will support better decision-making.
- Enhanced Fraud Detection Capabilities: As machine learning algorithms evolve, their ability to detect and prevent fraudulent activities will become more sophisticated, offering robust protection from financial losses and compliance risks.
- Real-Time Decision Support: Automated systems will provide finance teams with actionable insights in real-time, enabling them to identify transaction patterns, anomalies, and trends quickly, leading to more informed decisions.
- Seamless Integration with ERP and Financial Systems: Reconciliation tools will seamlessly integrate with ERP and financial systems, creating a unified ecosystem that streamlines operations and enhances overall financial management.
- Greater Focus on High-Value Financial Analysis: By automating routine tasks, finance teams will have more time to focus on higher-value activities, such as strategic planning, financial forecasting, and budgeting, which contribute to long-term business growth.
Conclusion: AI Agents for Transaction Reconciliation Process
In conclusion, AI agents are transforming the transaction reconciliation process, making it faster, more accurate, and more efficient. By automating routine tasks, minimizing errors, and providing real-time insights, AI empowers finance teams to achieve greater productivity and focus on strategic decision-making. With the integration of advanced technologies like machine learning, NLP, and RPA, the future of AI-driven transaction reconciliation looks promising, paving the way for smarter and more agile financial operations.